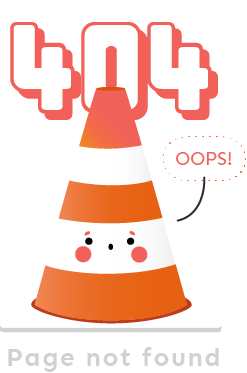
It seems we can't find what you're looking for. Perhaps searching can help.
thrive-automator
domain was triggered too early. This is usually an indicator for some code in the plugin or theme running too early. Translations should be loaded at the init
action or later. Please see Debugging in WordPress for more information. (This message was added in version 6.7.0.) in /home/dh_jg66yf/sturoberts.me/wp-includes/functions.php on line 6121It seems we can't find what you're looking for. Perhaps searching can help.